Get Started Today |
Introduction
As businesses scale generative AI (GenAI) systems from POCs into production, new challenges—often termed “day two issues”—emerge. These include infrastructure scalability, cost control, and operational complexity, as outlined in this Infoworld article. Beyond technical hurdles, continuous tuning, monitoring of outputs, and strong governance frameworks are essential to ensure GenAI systems remain aligned with business objectives, risk management, and compliance requirements.

Scaling Challenges and Operational Complexity in GenAI
As organizations scale their generative AI (GenAI) deployments, they encounter a variety of “day two” challenges that demand careful attention to ensure operational efficiency. One of the most common issues is latency. As data volumes increase and more users interact with the system, the process of retrieving relevant information from large datasets can slow down. This is especially problematic for retrieval-augmented generation (RAG) systems, which rely on external data sources to generate contextually relevant responses.
In RAG deployments, a key challenge lies in ensuring that the system can efficiently manage vector search processes, which match user queries to relevant data chunks stored in vectorized formats. Without optimization, RAG systems can suffer from vector search inefficiencies, leading to slower responses and a degraded user experience. Latency often comes from how long it takes to query the vector database and retrieve the correct chunks of data for processing by the large language model (LLM).
To mitigate these performance issues, several strategies can be employed:
- Caching frequent queries: Storing responses to frequently asked questions or similar queries reduces the need for full searches and can significantly decrease response times.
- Optimizing chunking and embeddings: Careful selection of how data is split into chunks and how it is embedded into vectors for storage can greatly improve retrieval speeds. Large or inefficient chunking can slow down searches and result in less relevant data being passed to the LLM.
- Cloud resource optimization: Scaling infrastructure in the cloud to handle peak demand is crucial, but so is optimizing the architecture to avoid over-provisioning, which can inflate costs without improving performance.
Ultimately, addressing these operational issues is about balancing speed, cost, and user satisfaction. Organizations must continuously refine their infrastructure and approach to ensure their GenAI deployments scale smoothly as user numbers grow.
The Importance of Measuring and Reviewing Outputs
Tackling day two challenges in generative AI deployments is not solely about optimizing infrastructure; it also involves ensuring that the system’s outputs remain relevant, accurate, and aligned with the organization’s goals over time. Measuring and reviewing outputs regularly becomes critical as models evolve, datasets change, and user interactions increase.
One of the most pressing risks is AI drift. This occurs when the model’s performance deteriorates due to outdated training data or new contexts emerging that were not originally considered during the model’s development. Left unchecked, AI drift can result in reduced accuracy, biased outputs, and even regulatory compliance issues.
To maintain the effectiveness of GenAI models, organizations should:
- Monitor outputs continuously: Automated tools should be implemented to track key performance indicators, such as accuracy, relevance, and user satisfaction with AI-generated responses. This ensures that outputs consistently align with business objectives.
- Retrain models regularly: As business needs evolve, the AI models must also be retrained with updated data to maintain their relevance. Regular retraining minimizes drift and keeps the system aligned with real-world changes.
- Establish feedback loops: Integrating a feedback mechanism where users can assess AI-generated responses helps refine the model in real time. This ensures that the system can learn from its mistakes and continuously improve the quality of its outputs.
By incorporating these strategies, businesses ensure that their GenAI systems remain valuable and reliable, providing outputs that contribute meaningfully to their objectives.
Managing Risk and Governance
In generative AI deployments, ensuring robust governance is critical to managing both operational risks and broader ethical, legal, and compliance requirements. Governance frameworks ensure transparency in how AI models make decisions, while also providing a structure for mitigating the risks inherent in AI outputs, such as bias, inaccuracies, or privacy violations.
Regular audits of AI systems can help organizations maintain control over the system’s decisions and outcomes. These audits ensure that the AI operates within the parameters defined by the business and regulatory bodies, and they are key to spotting biases or errors early. For industries bound by strict data privacy regulations, governance frameworks must ensure that the AI adheres to compliance standards like GDPR or HIPAA, which require stringent data handling protocols.
Governance is not just about compliance; it also encompasses the need for cross-functional alignment.
It is critical that teams from IT, legal, compliance, and business units collaborate on AI initiatives to ensure that risks are understood and managed from multiple perspectives. This ensures that the AI’s outputs align with both the organization’s ethical standards and its business goals.
Finally, transparency is a key component of governance. Organizations must ensure that their AI systems are explainable and that decisions made by the AI can be traced back to specific datasets and algorithms. This level of transparency helps build trust in the system, both internally and externally, and makes it easier to respond to any issues that arise.
The Role of Lifecycle Management
Managing the lifecycle of generative AI systems is an ongoing process that requires flexibility and a proactive approach to model maintenance. A common misconception is that once a generative AI solution is deployed, the heavy lifting is over. However, keeping a GenAI system running effectively requires constant fine-tuning and updates, especially as business needs and data evolve.
Lifecycle management involves regular model updates and retraining. AI models are trained on specific datasets at a given point in time, but as data grows and changes, the AI needs to be updated with new information. Regular retraining ensures that the AI system stays current and continues delivering relevant outputs. Businesses must establish processes for updating both the models and the data on which they rely.
In addition to model updates, businesses should take a stack-based approach to technology. This involves selecting tools and frameworks that can easily integrate new features and accommodate future requirements without requiring a complete overhaul. By focusing on microservices and modular architectures, organizations can add functionality, scale components, or replace tools as needed without introducing unnecessary complexity or operational risk.
Finally, lifecycle management includes the ongoing task of cost optimization. As the system grows, so too will its resource demands. By regularly reviewing how the AI system operates and where inefficiencies may lie, businesses can cut costs without sacrificing performance.
A well-managed AI lifecycle keeps the system adaptable, relevant, and efficient over time, ensuring long-term success.
Conclusion
Overcoming day two challenges in generative AI requires more than just scaling infrastructure—it demands a strategic focus on continuous tuning, monitoring, and governance. As the system evolves, organizations must ensure that AI outputs remain relevant, accurate, and aligned with their broader objectives. Regularly reviewing performance, managing risks, and embedding governance frameworks are crucial for maintaining quality and compliance. By implementing strong oversight across the entire AI lifecycle, businesses can not only mitigate risks but also maximize the long-term potential and value of their GenAI solutions.
This approach goes beyond addressing technical bottlenecks, pushing organizations to adopt holistic solutions that ensure both operational efficiency and strategic alignment. Continuous refinement of data processing techniques, such as vector searches and chunking methods, ensures that AI models remain responsive and relevant as data grows. Additionally, embedding governance and monitoring processes into AI operations strengthens transparency and accountability, which are crucial for maintaining trust with stakeholders and ensuring regulatory compliance.
Incorporating a lifecycle perspective further ensures that GenAI solutions can adapt to evolving business needs, integrate new functionalities with minimal disruption, and manage costs effectively. This means leveraging a stack-based approach that allows for modular updates and minimizes long-term operational overhead. By embracing flexibility and scalability at every stage, organizations can future-proof their AI deployments.
Ultimately, addressing these day two challenges empowers businesses to fully harness the potential of generative AI. By aligning technical performance with governance and risk management, companies can ensure that their GenAI systems continue to deliver both immediate value and long-term strategic benefits.
Acacia Advisors Can Help
The future is not on the horizon; it’s here, and it’s powered by AI. As you stand at this pivotal juncture, the decisions you make today will define your organization’s trajectory for years to come. It’s time to take the reins, to harness the transformative power of AI, and to drive your business towards unprecedented growth and success.
Partner with Acacia Advisors
We at Acacia Advisors invite you to take that crucial first step on your AI journey with a partner that understands the landscape and has the expertise to navigate it. Partnering with us means embarking on a path of transformation that is intelligent, responsible, and tailored to your unique business needs.
Discover the Potential
Visit chooseacacia.com to uncover the possibilities of a tailored AI strategy that can propel your organization forward.
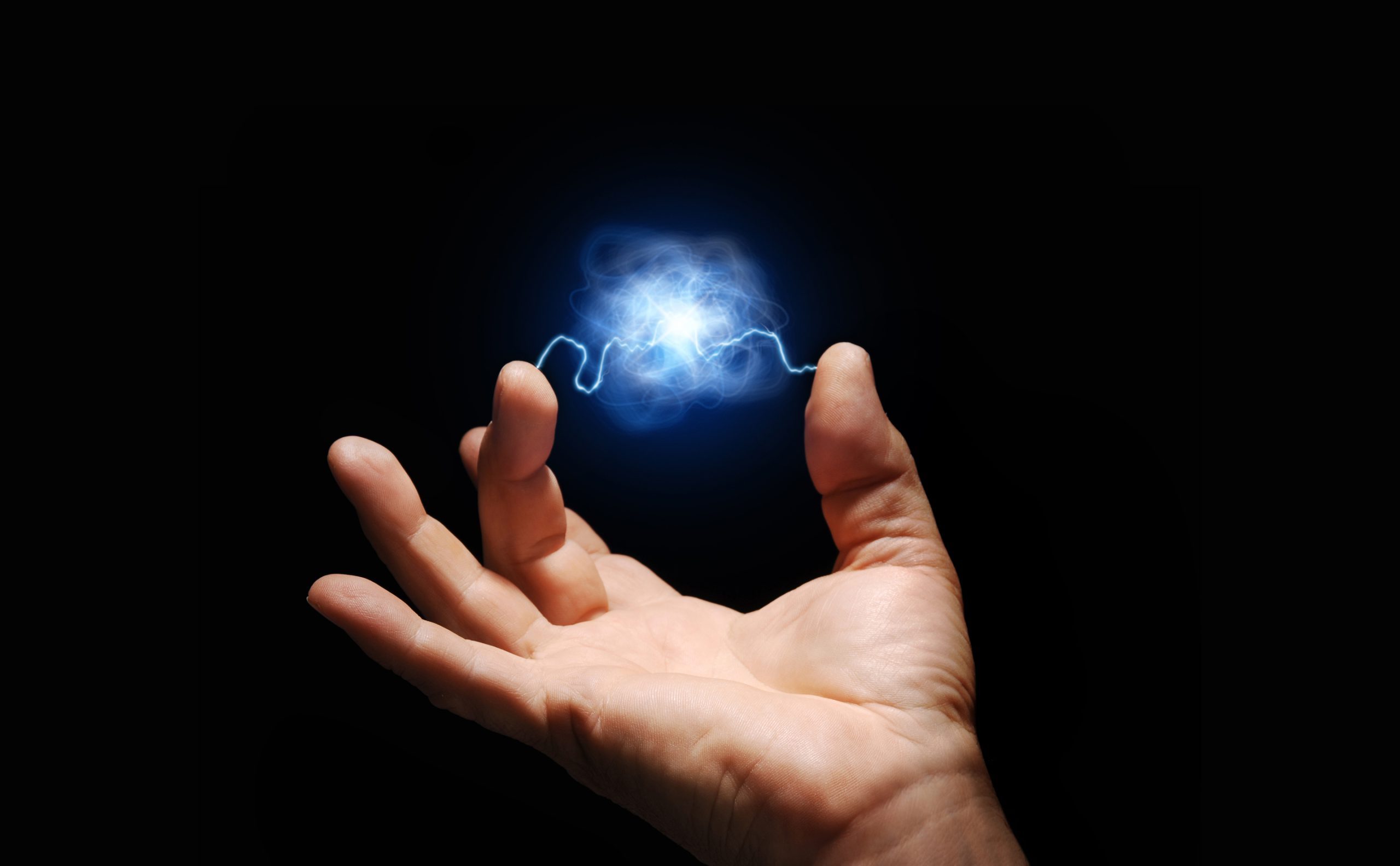
Begin Your Transformation Journey
Don’t let another moment pass in inaction. Contact Acacia Advisors today and lets start the conversation. Let’s explore together how to unlock new value for your business, enhance your operational efficiencies, and give you the competitive edge that you need in this digital age.
Your journey towards AI excellence starts with a simple step. Take that step with Acacia Advisors.
Contact Us Now
Embrace AI with confidence. Embrace it with Acacia Advisors.