Get Started Today |
From the blog series “Navigating the AI Revolution: Strategies for Success” by Acacia Advisors on AI Strategy (9 of 10)
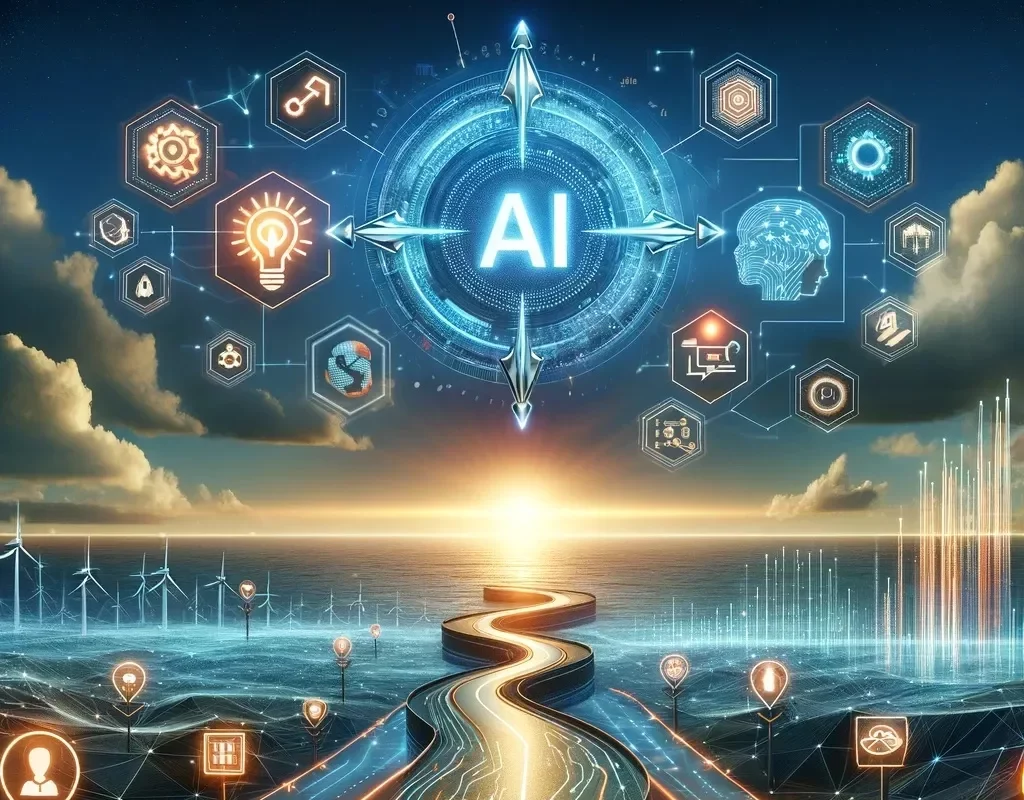
Introduction
In the rapidly evolving landscape of artificial intelligence (AI), the ability to measure success accurately is not just beneficial—it is imperative. As organizations invest heavily in AI technologies, establishing a clear and quantifiable measure of effectiveness ensures that these initiatives are not just innovative experiments but strategic investments aligned with core business objectives. The use of precise metrics and key performance indicators (KPIs) is essential to validate the impact of AI, guide future enhancements, and justify continued or increased investment in these technologies.
The Importance of Metrics in AI Initiatives
Measuring the success of AI initiatives is critical for several reasons. Firstly, it allows organizations to determine whether AI implementations are meeting their intended goals, such as improving efficiency, increasing sales, or enhancing customer satisfaction. Without concrete metrics, it’s challenging to ascertain the value AI is adding and difficult to make informed decisions about scaling up or optimizing AI systems.
Secondly, well-defined metrics provide a framework for accountability. They help set expectations for what AI should achieve and offer a means to assess performance against these benchmarks. This is particularly important in justifying the allocation of resources towards AI projects, as stakeholders want to see a return on their investment.
Lastly, metrics facilitate continuous improvement. By regularly measuring the performance of AI systems, companies can identify areas for enhancement, uncover new opportunities for application, and avoid stagnation in a technology landscape that is constantly advancing.
Setting the Context with KPIs and Metrics
Key Performance Indicators (KPIs) and other relevant metrics serve as the cornerstone for gauging AI effectiveness across various business functions. KPIs are quantifiable measurements that reflect the critical success factors of an AI initiative. They might include operational metrics such as speed and accuracy of task completion, financial metrics like ROI and cost savings, or customer-related metrics such as satisfaction scores and engagement rates.
Choosing the right KPIs involves understanding the specific objectives of each AI project and aligning those objectives with broader business goals. It’s not just about tracking any data available, but about tracking the right data that provides meaningful insights into how well the AI applications are performing.
In this blog post, we will delve deeper into the types of metrics that are most useful for AI projects, how to select the appropriate KPIs, and how to interpret these indicators to make informed strategic decisions. By the end, businesses should have a clear roadmap for measuring and maximizing the success of their AI initiatives, ensuring these technologies contribute positively and substantially to their overarching goals.
Understanding AI Success Metrics
To effectively measure the success of AI initiatives, it is crucial to understand the different types of metrics that can be employed. These metrics can broadly be categorized into efficiency, accuracy, performance, and financial impact. Each category serves a unique purpose and provides insights into various aspects of AI effectiveness. Selecting the right metrics is a strategic process that depends on the specific goals and the nature of each AI project.
Types of AI Metrics
- Efficiency Metrics: These metrics assess how AI technologies streamline operations and reduce the time and resources required to complete tasks. Common efficiency metrics include throughput (the amount of work processed in a given time), resource utilization rates, and the reduction in human intervention for automated processes.
- Accuracy Metrics: Particularly vital in projects involving data processing, prediction, and categorization, accuracy metrics measure the correctness of outputs provided by AI systems. For instance, in a machine learning model used for credit scoring, accuracy metrics would evaluate the percentage of predictions that correctly assessed the creditworthiness of applicants.
- Performance Metrics: These encompass a broader set of indicators that assess the overall effectiveness of AI applications. Performance metrics might include system uptime, response times, error rates, and the quality of user interactions with AI systems, such as chatbots or virtual assistants.
- Financial Impact Metrics: These metrics quantify the economic benefits derived from AI initiatives. They include return on investment (ROI), cost savings, revenue generated from AI-enhanced products or services, and overall financial contributions to business operations.
Selecting the Right Metrics
Choosing the appropriate metrics for an AI project requires a clear understanding of the project’s objectives and the specific challenges it aims to address. Here’s how to select the right metrics:
- Align Metrics with Business Objectives: Start by clearly defining what the AI project is supposed to achieve. If the goal is to enhance operational efficiency, then efficiency metrics will be most relevant. If improving customer satisfaction is the aim, then metrics related to customer engagement and satisfaction should be prioritized.
- Consider the Nature of the AI Project: The type of AI technology implemented (e.g., machine learning models, natural language processing applications) will also influence which metrics are most appropriate. For instance, projects involving predictive analytics will require strong emphasis on accuracy and performance metrics.
- Balance Leading and Lagging Indicators: Include both leading indicators (which predict future performance) and lagging indicators (which provide insights after an event has occurred) to get a comprehensive view of AI effectiveness. For example, the number of AI-driven interactions can be a leading indicator for customer engagement, while customer satisfaction scores are a lagging indicator.
- Benchmark Against Industry Standards: Where possible, compare chosen metrics against industry standards or benchmarks to gauge how your AI initiatives stack up against competitors and industry best practices.
By carefully selecting and applying these metrics, organizations can not only track the immediate impacts of their AI initiatives but also gain insights into how to enhance their long-term strategy. This approach ensures that AI deployments are not just technologically successful but also align closely with broader business goals, driving meaningful improvements across the organization.
Essential KPIs for AI Projects
To effectively gauge the success and impact of AI initiatives, it is crucial to track a set of well-defined Key Performance Indicators (KPIs). These KPIs should cover various aspects of business operations, from operational efficiency to customer satisfaction and revenue growth. Here’s a breakdown of essential KPIs that can help organizations measure the efficacy of their AI projects across these critical areas.
Operational Efficiency
Improving operational efficiency is often a primary goal of AI projects. The right KPIs can help quantify how AI is enhancing business processes:
- Process Times: This KPI measures the time taken to complete specific operations or processes before and after AI integration. A reduction in process times can indicate increased efficiency due to AI automation.
- Error Rates: AI is often implemented to reduce human errors in processes such as data entry, calculations, and transaction processing. Tracking error rates before and after AI implementation can highlight improvements in accuracy.
- Automation Levels: This metric quantifies the extent of tasks or processes automated by AI, typically expressed as a percentage of total operations. High automation levels can significantly reduce the workload on human employees, allowing them to focus on more strategic tasks.
Customer Satisfaction
AI-enhanced tools are increasingly used in customer service to improve engagement and satisfaction. Relevant KPIs include:
- Response Times: In customer service, faster response times can lead to higher customer satisfaction. AI can help minimize these times by automating responses and aiding human agents with quicker data retrieval and decision support.
- Service Quality: Measured through customer surveys, service quality KPIs assess the effectiveness of AI tools in meeting customer needs. High-quality service is often reflected in positive feedback and low complaint rates.
- Customer Retention Rates: Retention rates can be significantly impacted by the quality of service. AI’s ability to provide personalized experiences and proactive service can enhance customer loyalty and retention.
Revenue Growth
AI initiatives can also drive revenue growth by enhancing sales processes and marketing efforts. Key metrics in this area include:
- New Leads Generated: AI tools can help identify and nurture potential leads more effectively than traditional methods. Tracking the number of new leads generated through AI-powered campaigns can demonstrate the impact of AI on sales funnel expansion.
- Upsell Rates: AI’s capability to analyze customer data and predict buying patterns can be used to increase upsell opportunities. Monitoring upsell rates before and after deploying AI can show how effectively AI contributes to sales growth.
- Contribution to Sales: This KPI measures the percentage of total sales directly attributable to AI initiatives, such as recommendations made by AI systems or automated marketing campaigns. It helps quantify the direct financial benefits of AI to the business.
By tracking these essential KPIs, organizations can not only monitor the immediate benefits of their AI projects but also strategically refine their approaches to maximize long-term gains. These metrics provide critical insights that help stakeholders understand the value AI is adding, thereby supporting continued investment and expansion of AI capabilities within the enterprise.
Quantifying AI’s ROI
For business leaders, the decision to invest in AI hinges significantly on the ability to demonstrate a clear return on investment (ROI). Quantifying the ROI of AI initiatives involves a comprehensive analysis of both the tangible and intangible benefits AI brings to an organization, balanced against the costs incurred during its implementation. This section outlines how to effectively calculate and present AI’s ROI, ensuring that business leaders can make informed decisions based on solid financial and strategic insights.
Cost Savings vs. Investment Costs
Calculating the ROI of AI projects requires a detailed assessment of both the direct and indirect costs and savings associated with these initiatives:
- Direct and Indirect Costs: Start by compiling all costs related to the development and deployment of AI systems. This includes upfront expenses like software acquisition, hardware investments, and costs of integrating AI into existing systems. Additionally, consider ongoing costs such as maintenance, upgrades, training employees to use AI tools, and potential increases in operational costs due to new technology.
- Operational Cost Savings: AI often leads to significant reductions in operational costs. This can be quantified by measuring the decrease in labor costs due to automation, lower error rates leading to fewer reworks, and enhanced efficiency in processes that reduce resource consumption. Collect data before and after AI implementation to clearly demonstrate these savings.
- Revenue Enhancements: Besides cost savings, AI can drive revenue growth through various channels. Increased sales from AI-enhanced marketing, higher customer retention rates due to improved service quality, and new revenue streams from AI-driven products or services are critical to consider. These should be quantified to the extent possible and included in the ROI calculation.
Calculating ROI: The ROI can be calculated using the formula:

This formula provides a percentage that reflects the return on every dollar invested in AI, considering both savings and additional revenue.
Long-term Value Creation
Beyond immediate financial metrics, it’s crucial to consider the long-term strategic benefits of AI:
- Innovation Stimulation: AI technologies often lead to new ways of working, enabling companies to innovate more effectively. The ability to quickly analyze vast amounts of data can lead to discoveries that keep companies at the forefront of their industries.
- Competitive Advantage: Implementing AI can provide a significant edge over competitors. This advantage may come through superior customer insights, better quality products, or more efficient service delivery. Quantifying this advantage can be challenging but is essential for understanding AI’s full impact.
- Scalability: AI systems can scale with your business, providing long-term value as they adapt to growing data volumes and complexity. This scalability ensures that investments in AI continue to pay dividends well into the future.
- Enhanced Decision-Making: AI’s ability to provide real-time insights and predictive analytics can vastly improve decision-making processes. While hard to quantify, this strategic benefit can lead to significant market advantages and risk mitigation.
In conclusion, when quantifying AI’s ROI, it is vital for business leaders to incorporate both immediate financial returns and broader strategic benefits. This comprehensive approach will not only justify the current investment but also pave the way for future AI initiatives, ensuring sustained growth and innovation in an increasingly competitive landscape.
Challenges in Measuring AI Success
Accurately measuring the success of AI initiatives is critical for validating their impact and guiding future investments. However, this task is often complicated by several inherent challenges, particularly related to data management and the dynamic nature of business environments. Addressing these challenges is essential to ensure that AI metrics and KPIs provide reliable and actionable insights.
Data Complexity
One of the primary obstacles in measuring AI success is the complexity of data involved. AI systems require large volumes of data to train and operate, and the quality of this data can significantly affect the outcomes of AI applications.
- Data Quality: Poor data quality is a common issue that can skew AI performance and lead to inaccurate conclusions about its effectiveness. Inconsistencies, incomplete data sets, and erroneous entries all undermine the integrity of AI outputs. Ensuring data cleanliness and accuracy is paramount for reliable measurement.
- Data Consistency: AI systems often pull data from multiple sources, which may not always be synchronized or standardized. Differences in data formats or updates can lead to inconsistencies that complicate analysis and performance tracking. Establishing rigorous data integration and preprocessing standards is crucial to mitigate these issues.
- Data Accessibility: Sometimes, the data needed to measure AI success is not readily accessible due to privacy concerns, regulatory restrictions, or technical barriers. Developing a framework that ensures data is accessible while complying with all legal and ethical guidelines is necessary for ongoing AI evaluation.
Dynamic Environments
AI systems do not operate in a vacuum; they are deployed within ever-changing internal and external business environments that can significantly impact their performance and the relevance of the metrics used to measure their success.
- Internal Changes: Organizational changes such as updates to IT infrastructure, changes in management policies, or shifts in strategic direction can alter the effectiveness of AI systems. For instance, a new IT policy might restrict the data AI systems can access, reducing their effectiveness or skewing performance metrics.
- External Fluctuations: Economic shifts, technological advancements, and competitive dynamics can also influence AI performance. An economic downturn might change consumer behavior patterns, affecting the predictions of AI models in marketing analytics. Similarly, new technologies may render existing AI tools obsolete or less effective.
- Adapting Metrics to Change: To maintain the reliability of AI measurements, it’s necessary to regularly review and adjust KPIs and metrics to reflect current realities. This adaptive approach ensures that evaluations remain relevant and that AI systems continue to meet organizational needs even as conditions change.
Strategies for Overcoming Measurement Challenges
Implementing robust data governance practices is essential to address data complexity issues, ensuring high-quality, consistent, and accessible data for AI systems. Additionally, building flexibility into the AI measurement framework allows businesses to adapt metrics and KPIs in response to internal and external changes. Regular audits and updates to the measurement framework can help mitigate the impact of these dynamics on the evaluation of AI success.
By acknowledging and addressing these challenges, organizations can improve the accuracy and reliability of their AI success measurements, making these insights truly actionable for decision-making and strategic planning.
Conclusion
The integration and scaling of AI within an organization bring transformative potential, but the true measure of this technology’s value lies in its tangible results. This is why the meticulous measurement of AI’s impact through well-defined key performance indicators (KPIs) and metrics is indispensable. These tools not only quantify the success of AI initiatives but also guide strategic decision-making and future technology deployments. As we conclude, let’s recap the critical role of these metrics and the value they bring to any organization investing in AI.
Demonstrating AI’s Business Value
The primary value of implementing precise KPIs and metrics lies in their ability to demonstrate the concrete business benefits of AI technologies:
- Quantifying Performance Improvements: Metrics such as process efficiency, error reduction, and response times provide clear, quantitative data that showcases how AI improves operational workflows and outputs.
- Validating Financial Returns: Financial impact metrics, including ROI, cost savings, and revenue enhancements, directly link AI initiatives to the bottom line. These figures are crucial for justifying ongoing and future investments in AI technologies.
- Enhancing Customer Experiences: Customer-centric metrics help quantify how AI tools improve service delivery, customer satisfaction, and retention, which are vital for competitive differentiation.
Guiding Future AI Strategies
Beyond their role in validation, KPIs and metrics are invaluable for refining and guiding future AI strategies:
- Strategic Alignment: Metrics ensure that AI initiatives remain aligned with broader business objectives, providing a benchmark against which strategic adjustments can be made.
- Identifying Opportunities for Improvement: Continuous measurement helps identify underperforming areas and opportunities for enhancement, ensuring that AI systems evolve in response to operational needs and market conditions.
- Facilitating Scalability: By monitoring success at various scales, metrics can inform decisions on whether and how to expand AI applications across the organization.
Future Planning
The dynamic nature of AI technology and its applications means that the metrics used to measure it must also evolve. Keeping your KPIs and metrics up-to-date ensures that they remain relevant and continue to provide valuable insights. This adaptive measurement approach supports sustained innovation and growth, ensuring that AI continues to deliver strategic value in the long term.
Acacia Advisors’ Role
Acacia Advisors remains committed to guiding organizations through the complexities of AI measurement. With our expertise in analytics and strategic planning, we help ensure that your AI initiatives are not only measurable but also maximally beneficial. By partnering with us, you can rest assured that your investment in AI is continually optimized, well-justified, and aligned with your evolving business needs.
In conclusion, the meticulous measurement of AI through strategic KPIs and metrics is not just beneficial—it is essential for maximizing technology investments. These tools provide the necessary data to demonstrate value, make informed decisions, and strategically scale AI implementations, ensuring that your organization reaps the full benefits of its digital transformation efforts.
The Role of Acacia Advisors in AI Measurement
Accurately measuring the success of AI initiatives is a complex but critical endeavor for any organization looking to leverage artificial intelligence strategically. Acacia Advisors is adept at guiding businesses through this intricate process, providing expert analytics support, customized metrics and dash-boarding, and strategic guidance. Our approach ensures that AI measurement frameworks are not only robust but also adaptable to the unique needs and evolving conditions of your business.
Expert Analytics Support
Acacia Advisors offers comprehensive analytics support to help organizations develop and implement effective frameworks for measuring AI success. This support includes:
- Framework Development: We assist in creating comprehensive measurement frameworks that are aligned with your organization’s strategic goals and the specific objectives of each AI initiative. These frameworks are designed to capture all relevant data points and provide a holistic view of AI performance.
- Data Integrity Solutions: Recognizing the challenges associated with data complexity, our team works to ensure that your data is clean, consistent, and comprehensive. We implement data governance practices that enhance data quality and reliability, which are crucial for accurate AI performance analysis.
- Analytical Tool Integration: Acacia Advisors helps integrate advanced analytical tools that automate data collection and analysis processes. These tools provide continuous, real-time insights into AI performance, facilitating timely decision-making and adjustments.
Customized Metrics and Dashboards
Understanding that each department within an organization might have different needs and goals for AI, Acacia Advisors excels in creating tailored analytics solutions:
- Custom Metrics Development: We develop customized metrics that are specifically designed to measure the key aspects of AI performance relevant to different business units. This customization ensures that each department can track the most meaningful indicators of success.
- Interactive Dashboards: Our team designs and deploys interactive dashboards that provide at-a-glance insights into AI metrics and KPIs. These dashboards are user-friendly and can be customized to show the most pertinent data for various stakeholders, ensuring that everyone from team managers to C-suite executives can easily understand and act on the information presented.
Strategic Guidance
As market conditions and business strategies evolve, so too must the metrics used to measure AI success. Acacia Advisors provides ongoing strategic guidance to ensure that your AI measurement approaches remain effective and relevant:
- Adaptive Metrics Adjustments: We continuously monitor external and internal changes that could impact AI performance and adjust KPIs and metrics accordingly. This proactive approach ensures that your measurement frameworks remain aligned with current business realities.
- Strategic Review Sessions: Acacia Advisors conducts regular strategic review sessions with your team to reassess and refine AI measurement strategies. These sessions help to identify new opportunities for AI application, address emerging challenges, and recalibrate goals as necessary.
- Long-Term Planning Support: Beyond immediate metric adjustments, we help you plan for the long-term evolution of your AI strategies. This includes anticipating future technological advancements and market trends that could influence how AI should be measured and utilized.
In conclusion, the role of Acacia Advisors in AI measurement is to ensure that your organization not only has the tools to track AI success accurately but also the strategic support to adapt and thrive in an ever-changing technological landscape. With our expert guidance, you can maximize the value of your AI investments, making data-driven decisions that propel your organization forward.
Begin Your Transformation Journey
Don’t let another moment pass in inaction. Contact Acacia Advisors today and lets start the conversation. Let’s explore together how our AI strategy development services can unlock new value for your business, enhance your operational efficiencies, and give you the competitive edge that you need in this digital age.
Your journey towards AI excellence starts with a simple step. Take that step with Acacia Advisors.
Contact Us Now
Embrace AI with confidence. Embrace it with Acacia Advisors.
Disclaimer
“The use cases presented in this blog post are used for illustrative purposes only. These examples serve to demonstrate the broad potential and value of artificial intelligence across various industries. Please note that these specific use cases were not directly delivered by Acacia Advisors but are highlighted here as industry examples.”